We are actively seeking industry, defense, manufacturing, national laboratory, or foundation partnerships to expand and improve our programs. We have a wide range of research across the center, presented below, that may serve as a opportunities as the basis for veteran fellows and veteran research assistants.
We are specifically looking for entities that are interested in:
- Direct funding of student veteran fellows (VetIF) or research assistants (VetRA)
- Summer interns from our Student Veteran pool
- Access to our eight university wide veteran talent pool (>3000 military and veteran students)
- Sponsored research projects centered on the inclusion of student veterans
- Any combination of interactions that may enrich the program, the partner organization, and the experience of our student veteran researchers.
If interested, please reach out to the VetIN Program Manager, Dr. Jeff Raymond ([email protected])!
Examples of Center Research for the Training of Student Veteran Research
Predictive 3D Printing of Composites
Researchers: Larson Group (UM) and Dow
Utilizing four distinctive rheological measures, as well as engineering controls like nozzle shear rate, this collaboration has provided a clear path to predicting form retention in 3D printed silicone composites. This was done by defining and testing recoverable yield stress, creep yield stress, flow stress, and printing yield stress. Ongoing work is being done to generate a general theory of print form retention with a variety of composites.

Electrochemomechanical Design Rules for Next-Generation Batteries
Research Teams: Qian Group (UIUC)
Our team is utilizing cutting-edge 4D electron microscopy to assess, characterize, and predict the electronic performance of next generation battery application materials. Using particle-level microstructure complexity at the cathode-particle and particle-electrolyte interfaces, we are working towards full-stack battery performance prediction based on our ability to tune defect and network structures. This work is founded on our ability to assess thousands of short- and long- range additive-particle interactions simultaneously in order to develop statistical design rules for tuning electrochemomechanical responses. We are seeking industrial partners to take our approaches and models to larger scales in verticals that need mechanically robust next-generation batteries.
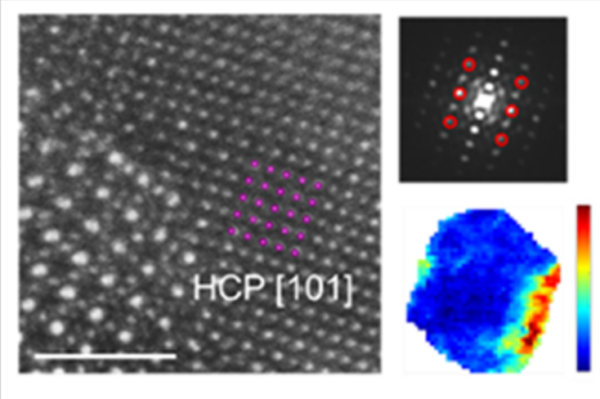
Advanced Coating from Hedgehog Particles
Research Team: Kotov Group (UM)
Using biomimetic approaches and self-assembly, we are able to produce functional additives with exceptional flexibility in composition, surface area, and responsiveness. Our hedgehog particles (HPs) are scalable and can be made from dozens of elements and be produced to express any organic material on their surfaces for incorporation into any solvent system or cure chemistry. Regardless of application, we have shown the ability to tailor these systems for high charge transport, phenomenal surface activity, exceptional surface areas, high temperature stability, and chemical resistivity. Additionally, we are able to tune optoelectronic and photonic response to a variety of wavelengths (IR, NIR, visible, and UV). We are seeking industrial partnerships that will allow us to transfer these scalable, tunable, and green material systems to coating and paint applications.

Printing of Chiral Plasmonic Patterns onto Surfaces, Objects, and Fabrics
Research Team: Kotov Group (UM)
We have demonstrated the ability to rapidly print patterned plasmonic surfaces using circular polarized light. We achieve this bye enforcing a handedness onto materials while printing utilizing light-to-matter transfer of twist. The resultant prints can be encoded as patterns, printed onto both rigid and flexible parts and surfaces, and have exceptional plasmonic and chiro-optical responses. We are seeking industrial partnerships that will allow us to contribute to long range asset tagging, machine vision, optical polarization devices and screens beyond the abilities of LC technology, or flexible optoelectronic devices.

This material is based upon work supported by the National Science Foundation under Award No. 2243104.
Any opinions, findings and conclusions or recommendations expressed in this material are those of the author(s) and do not necessarily reflect the views of the National Science Foundation.